Siri, Alexa and unconscious bias: the case for designing fairer AI assistants
Voice-assistants are becoming a part of our everyday lives, but they don’t understand everyone’s voices equally. We explore how the industry is addressing that inequality.
“I have yet to meet the person who is designing to intentionally discriminate,” Miriam Vogel tells Design Week. “But sometimes it’s hard to admit that you’ve designed something that might have gaps and flaws in.”
Vogel is executive director of EqualAI, a US-based initiative that aims to prevent unconscious bias in artificial intelligence (AI) development. But when it comes to one of the most widespread uses of AI — voice-assistants — the machines themselves do discriminate.
Research shows that while a white American male has a 92% accuracy rate when it comes to being understood by a voice-enabled assistant, a white American female has a 79% accuracy rate. A mixed-race American woman only has a 69% chance of being understood. (The reason for the American bias is that the most popular assistants — Amazon’s Alexa, Google Home and Apple’s Siri — are all based in California.)
“The example of the voice assistant is so poignant because it’s becoming such an important part of our daily life,” Vogel says (last year, an estimated 3.25 billion voice assistants were in use around the world). “Yet it’s riddled with questions about what language the code if written in, what voices, accents and tones it can and can’t hear.”
“It’s a clue into the bigger system,” she adds. “If we continue to let AI be designed by a homogenous set of players without bringing in different voices and perspectives, our voice assistants won’t have the benefits of diverse vantage points.”
“Notoriously white male centric”
The problem can result in light-hearted miscommunication — particularly in Siri’s misunderstanding of accents. But as Vogel points out, if there’s a miscommunication in medical technology — an area where voice-enabled technology is becoming more important — it could have serious, even fatal consequences.
The problem lies in the development process: as few as 13.5% of workers in the machine learning field are female. And the datasets used for technology are taken from clinical trials which are “notoriously white male centric”, according to Vogel.
Vogel has experience in the field of workplace bias; she led the Equal Pay Task Force under President Obama which promoted equal pay for women. A lawyer by training, she also led the development of the Implicit Bias Training for Federal Law Enforcement. She believes that the approach to creating a more diverse AI is through a mix of perspectives, not just tech developers but also by convening law-makers, academics and business leaders.
EqualAI also runs workshops with tech companies to help them “follow best practices to avoid the infusion of implicit bias”. This is where it can get complicated, Vogel says. Explaining to designers that their creation might be flawed can lead to “defensiveness”. “Part of our work is to let people know that no one can be omniscient,” Vogel adds. “The flaw of being human is that you have to expect this is going to surface in your AI, so you need to plan around it.”
EqualAI also has a corporate focus: Vogel explains to companies that they are more likely to sell products that understand their potential customers. This “consumer education” is crucial to a product’s commercial success: “Can this voice recognition understand me, as a female? Can it understand my family’s voice back in China?”
Alexa, Siri, OK Google… Beeb?
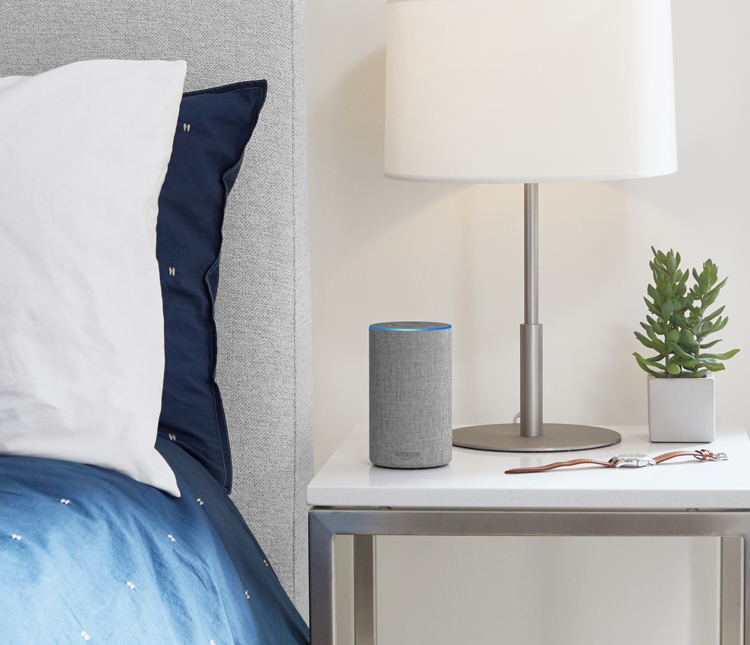
While the main players in this field are American, Vogel says that the British are “far ahead in their thinking” about bias in AI. (Some of EqualAI’s founding members are British including Wikipedia founder Jimmy Wales and business woman Martha Lane Fox.) And this year, the BBC is launching its own voice assistant, which will be known by the wake word — the name the user says to ‘wake’ the device — Beeb.
The BBC says it’s in a good position to create a British-focused voice-assistant. “People know and trust the BBC, so it will use its role as public service innovator in technology to ensure everyone — not just the tech elite — can benefit from accessing content and new experiences in this new way,” it says. It will work on voice-enabled devices.
The project is being headed up by Mukul Devichand, the executive editor for BBC voice and AI. Though the BBC’s digital team could not provide any more comment on the voice-enabled assistant yet, it has outlined how the project works. It is asking teams from offices around the UK to spend a few minutes recording their voices “to make sure everyone’s accent can be recognised when it launches”.
And while it will have a more diverse dataset — and likely more regional accents than an average Silicon Valley office — it is still part of the broadcaster’s commercial strategy. “It will also allow the BBC to be much more ambitious in the content and features that listeners can enjoy,” it says.
Towards a more diverse dataset
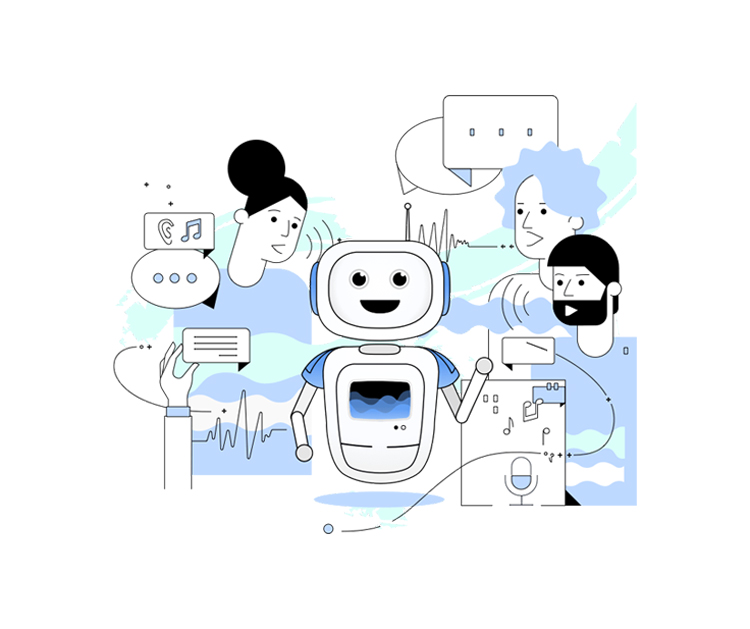
What might the BBC’s open source dataset look like? A comparison could be found in Mozilla’s Common Voice Project. Founded in 2017, the web browser’s project hopes to “speed up the process of collecting data in all languages throughout the world, regardless of accent, gender or age,” according to Mozilla’s head of machine leaning, Kelly Davis.
Davis says that tech giants have an advantage because of their “propriety access to voice data”. “They also tend to work better for men than women and struggle to understand people with different accents, all of which is a result of biases within the data on which they are trained,” he adds. (Questions around the storage and use of that data also “remain unanswered”, Davis points out.)
With volunteer consent, Mozilla crowdsources data collection in an attempt to “enable new voice-assisted technology that is much more accurate and representative of the global population”. People around the world “donate” their voices to a dataset that is then freely available to start-ups and companies who are developing voice-enabled technology.
The Common Voice dataset is now “the largest public domain transcribed voice dataset” in the world, with over 4,000 recorded hours of voice data and 35 languages including English, French, German and Mandarin. Contributors can also provide metadata about their age, gender, and accents so that their voices are tagged with “information useful in training speech regimes”.
Languages across the world have contributed from Welsh to Kabyle, an indigenous community in northern Algeria. This highlights another bias in voice technology: a focus on English, which is the most profitable language to design AI systems for, according to Davis.
A new development in the Common Voice project has been the inclusion of endangered languages. “It’s evolved from being a project to create open speech data sets for under-resourced languages to a project that also has a language preservation component,” Davis says, which he calls both “an honour and a burden”.
A more “diverse voice technology ecosystem”
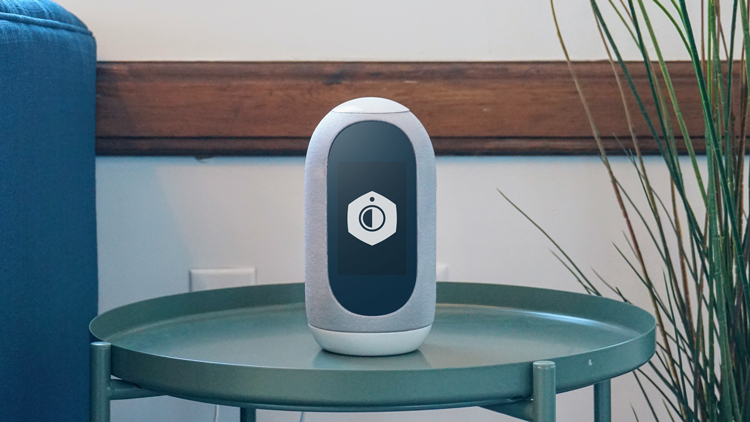
Crucially, Mozilla’s dataset is being used: by Mycroft (an open source voice assistant named after Sherlock Holmes’s elder brother), Te Hiku media (a New Zealand-based charitable media organisation), Iara Health (a Brazilian Portuguese medical transcription tool). Davis Says that Mozilla’s aim moving forwards is to contribute to a more “diverse and innovative voice technology ecosystem”. That means releasing voice-enabled products themselves, and “supporting researcher and smaller players”.
Last year, Mozilla partnered with the German Ministry for Economic Cooperation and Development to support “initiatives in Africa to collect local language data”. These datasets are to be used for voice-enabled products and technology that are “relevant” to the country’s Sustainable Development Goals.
With talk of a widespread voice-enabled future, how likely is it for an entire industry to rely on a single open source data project? As of 2020, there’s 38GB of data in the English language section of the dataset. And of people who have tagged their data, the split between men and women is 46% to 13%. If you were developing an app for Slovenians, you’d only be working with 175MB of voice data. There are clearly limits to a project powered by volunteers.
“An essential time for AI”
For EqualAI, progression comes through understanding the scope of AI. “It’s an essential time for AI and we see the harms, but we also see its power,” Vogel says. “The fun part of my job is to enlist people into being better humans — which they want to be. It’s about telling them how to create a better product.”
“That’s the carrot,” Vogel says, but it’s also essential that designers are mindful of very real downsides. “The stick is telling them that their product, company and brand reputation will suffer if they don’t take this challenge on — and by the way, there are lawyers making the case for liability and it’s growing as people start to understand this field better.”
-
Post a comment